Sent to you by nigel via Google Reader:
via The Science of Sport by noreply@blogger.com (Ross Tucker and Jonathan Dugas) on 1/5/09
The Matthew Effect: Talent ID and sports science applicationThe first post of 2009 is inspired by a book I read over the break - Outliers by Malcolm Gladwell. One of the very first things I recall learning when I started out my postgraduate studies was a tip from Prof Tim Noakes to read widely, and read outside your field. Sensible advice, and it informs much of what we are about here at The Science of Sport.
And in that light, Malcolm Gladwell's book, Outliers, provides the latest inspiration for a somewhat novel look at sports science and talent ID (it's "somewhat novel" because it's actually a well-established phenomenon, but has not been published in sports science journals, but mainly social science journals). Gladwell is not entirely outside my field, it must be said, because much of it deals with economics, marketing, strategy etc. But, nevertheless, it's a different perspective on sports science, especially when it covers such obvious territory as the topic of today's post.
That territory, for those of you who have read Outliers, is called The Matthew Effect.
The Matthew Effect - lessons from ice-hockey, soccer and rugby
Today's post is really just a summary of what is described in the book, with the addition of an example from South African rugby that I've managed to do so far, and my own interpretations. There is much more to come, however, including more examples (some of which I hope you'll provide), as well as discussion of how different sports might be affected by this phenomenon, what might be done, and what the implications are for sports science and management.
But for now, forgive me for merely summarizing the data provided in the book, and take a look at the following set of pie-charts. What you are looking at are the breakdown of month of birth of junior players in four different high level sports teams. From top left, going clock-wise: A Canadian Junior Champion team from 2007, the Czech Junior Football/Soccer team from 2007, the Czech Junior Ice-Hockey team from 2007, and the 2007 South African Schools Rugby team.
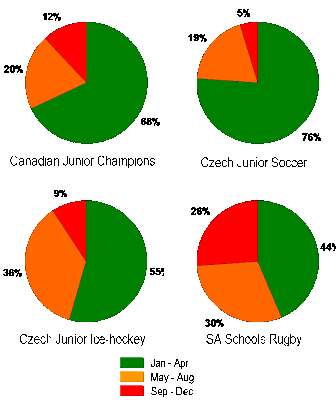
What should jump out is the enormously high percentage of high-level players who are born between January and April. All told, out of the 91 junior players making up the above four teams, 55 of them (60%) were born in the first four months of the year, and only 13% in the last four months.
This is not an isolated finding, and is true across just about any sport at a high-level. It was reportedly first observed in the mid-1980's by a Canadian psychologist named Roger Barnsley. He noticed that a disproportionately high percentage of high-level ice-hockey players were born in the first few months of the year, and almost none towards the end of it. He expanded his study and looked at other sports like football and baseball, and even started to examine the effect of birth-month on things like academic achievement, suicide and self-esteem. You can read some of those studies here.
The reason - relative age, and a confusion of ability with maturity
We know you're pretty sharp, so it will probably come as no great surprise to learn that this finding is the result of the effect of RELATIVE age on sports performance, and the very easy mistake that coaches are lured into making. If you thought that it was the result of their star signs and some astrology, then I'm afraid you were on the wrong track! But, you might enjoy this website a little more...
Back to reality, and the suggestion that the reason so many elite athletes are born in the early months of the year is the result of the very large effect that 10 months difference in age can have on young children's ability to play sport.
An example: Ross and Jonathan
Let's take two 10-year olds, Ross and Jonathan. They are both 10 years old on the first day of January 2009, and so they compete in the Under-11 age group of their sport (soccer, let's say).
However, not all 10-year olds are created equal! Ross is 10 years and 11 months old on the first day of 2009 (his birthday is in February). Jonathan is 10 years and 1 month old (his birthday is in December), and so he is a full 10 months YOUNGER than the people, like Ross, who he is going to compete against.
At the age of 11, when skills and strength, and the other attributes required for sporting success are still developing, 10 months is an eternity. Think back to your own development, or better yet, to the development of your children, if you have them. Backyard games of catch or football or rugby or cricket change dramtically from one year to the next because a child at that age acquires skills and strength so quickly that they improve enormously from one month to the next.
This means that the 10-month advantage that Ross has, by virtue of being born in January or February, will manifest itself as a big performance advantage over Jonathan (obviously, I'm generalising here, you'll find exceptions. But the graphs above suggest that they happen infrequently).
Enter the coach, and the Matthew Effect is born
Now the coach enters the picture. He has a team of energetic, uncontrollable young 10-year olds to look after, and he picks his team, and allocates his time and attention to those who are deemed to have the most potential. However, he is unable to distinguish between capacity for performance and maturity. Maturity determines ability - Ross is older, and may possess more strength, speed, skill and therefore appear the star player in practice. Jonathan is yet to develop these attributes, but may have the talent.
However, the fateful decision made by the coach is to pick Ross ahead of Jonathan. What happens next determines the distribution you see in the graphs above. By virtue of having been picked based on his "superior" ability, Ross plays against higher quality competition, receives better coaching, more attention, and therefore improves MORE than Jonathan will. Their paths are determined from the outset, based on their selection, and the different journeys they will take are going to mean that one day, Ross is the better athlete or player, thanks to these advantages and opportunities he has received.
At the same time, Jonathan is far less likely to continue to play, because:
- He is often smaller than the guys he competes against, and that's not likely to make his life much fun!
- He becomes discouraged at the ever-growing gap between himself and the other guys who are being more heavily invested in
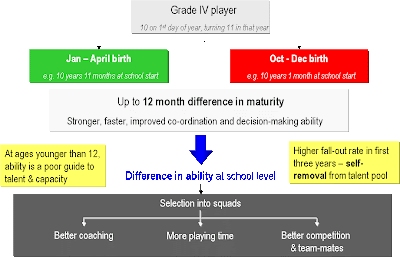
And why is it called the Matthew Effect, you may be asking? That name was coined by the sociologist Robert Merton, based on the bible verse from Matthew: "For everyone who has will be given more, and he will have an abundance. Whoever does not have, even what he has well be taken from him" (Matt 25:29).
That means that success comes to those that are successful, thanks to their advantage, in this case, from their relative age.
Confounders and debate
There are of course debates and issues around this. The presence of athletes who are born between September and December (albeit a low 13%) suggests that exceptions do exist. What might be very instructive is to examine how those "outliers" (apologies, Malcolm) reach the level they do - they may be early developers, they may have parents who start them out by playing games at a younger age developing their skills "ahead of the normal curve", or they may have older siblings who drag them to a higher level of performance despite their younger relative age. All these options are intriguing and instructive for talent ID purposes, and for understanding how sporting success is determined.
The three elements required: Selection, streaming and differentiated experience
To dig into the effect a little further, the afore-mentioned Roger Barnsley suggested that three things are required for this effect to occur:
- Selection - someone (in this case, a coach) must be selecting players based on ability
- Streaming - once selected, players are placed into streams. These can be competitions, teams, training squads etc.
- Differentiated experiences - very importantly, once in the streams, players receive different levels of coaching, competition and opportunity. This is summarized in the figure above.
The lack of a meritocracy can be seen in the slightly more balanced distribution of the SA schools rugby team, by the way. In that team, "only" 44% were born in the first three months of the year, much lower than for the other three teams. So what, you ask? Well, that's because the SA school team was selected out of a mix of "traditional" and "non-traditional" rugby schools. Without going into the history and politics of our SA rugby, we have some schools that are very heavily based around performance, and have multiple teams at each age group, right down to junior level. Others are not as focused on age-group performance, and because of the selection policies in SA sport, the team is a mixture of the two. This dilutes the Matthew Effect. You'll find that whenever merit is NOT the primary factor for selection, this will occur.
Implications for sports science
Well, I'm running out of time (and so are you, probably!). This post has run over-time, but there's much more discussion on this topic to come. One area that interests me in particular is that talent ID often does not note this potential confounder of age. It is for this reason that the identification of talent, especially if that result is going to be used to "stratify" young sportspeople into different paths, should occur as late as possible.
What this suggests is that a sports scientist who plans to do some talent ID on a group of unknown sports people might as well make his first selection on the basis of date of birth! OK, that's being too extreme, but the point is, by the time a sports scientist tests a squad of potential athletes aged say 19 years, it's often too late to undo the effect that he measures, and which may have been created 9 years earlier. More to the point, the sport in question might be missing out on some of its best potential talent, which was lost 9 years earlier thanks to a wrong selection! At the senior level, the coach who picks the team may believe he is picking from the whole pool, when in fact, 40% of it has been lost through premature selection, 9 years previously! That should be a significant "flag" for people involved in high performance sport...
More to come...
Ross
The Science of Sport Dr. Ross Tucker Dr. Jonathan Dugas
Things you can do from here:
- Subscribe to The Science of Sport using Google Reader
- Get started using Google Reader to easily keep up with all your favorite sites
No comments:
Post a Comment